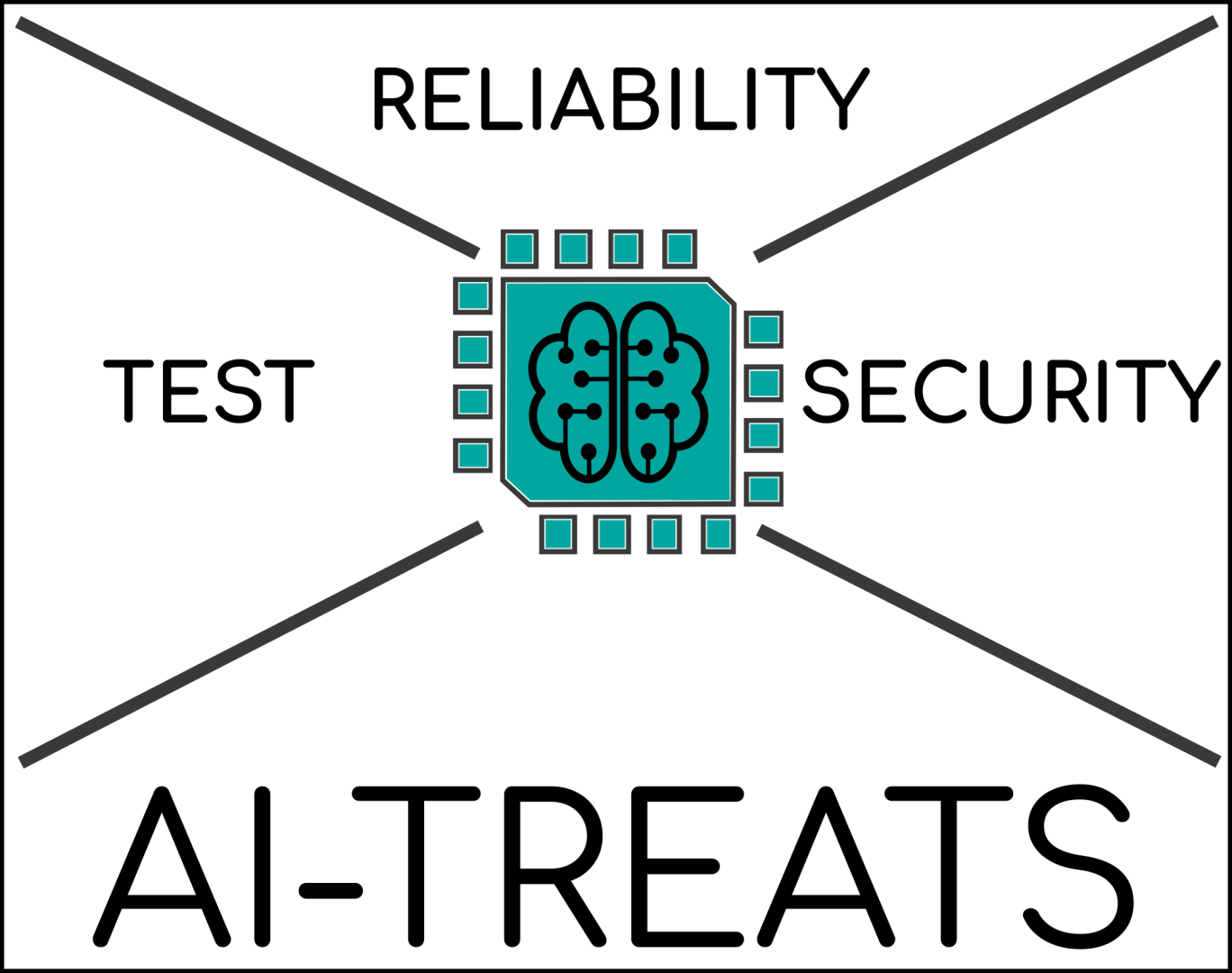
Recent advances in Artificial Intelligence (AI), particularly deep learning, have led to numerous applications, including computer vision, speech recognition, natural language processing, and robotics. Autonomous vehicles are also afoot, and AI will be the core enabling technology. In parallel, there is intense activity in designing dedicated hardware for AI. On the one hand, AI hardware accelerators are demanded to support the tremendous processing power, unprecedented speed, and memory costs that deep neural networks require so as to realize their full potential. On the other hand, there is a large incentive for moving the AI algorithms execution from the cloud into the edge devices, i.e., Internet-of-Things (IoT) devices, in particular for meeting data confidentiality and network bandwidth requirements and eliminating communication latency. Edge devices are expected to include local AI processing, yet this is challenging as an edge device is in a resource-constrained environment. AI hardware design efforts rapidly evolve, exploring various architectures (e.g., machine learning-based, spiking), design flavors (e.g., digital, mixed analog-digital), and emerging technologies (e.g., memristive devices arranged into crossbars to implement efficiently the multiply-add matrix operations in neural networks).
The aim of this Workshop is to focus particularly on the following emerging problems pertaining to AI hardware:
•Testing: fault modeling, fault simulation, test generation, post-manufacturing testing, design-for-test, built-in self-test, online testing, fault diagnosis.
•Reliability: reliability analysis, design-for-reliability, fault-tolerance, self-repair, functional safety.
•Hardware security and trust: IP/IC piracy, hardware Trojans, side-channel attacks, fault injection attacks.